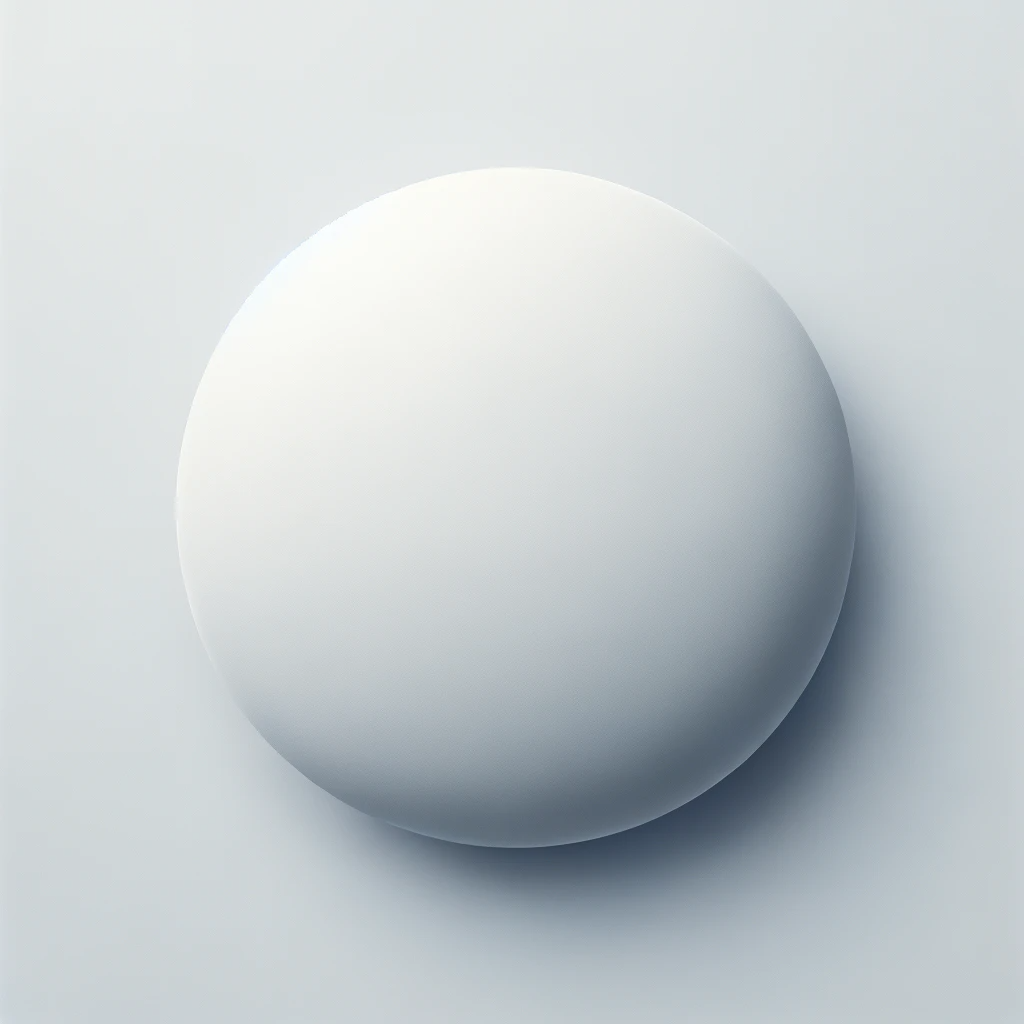
In order to be able to cluster text data, we’ll need to make multiple decisions, including how to process the data and what algorithms to use. Selecting embeddings. First, it is necessary to represent our text data numerically. One approach is to create embeddings, or vector representations, of each word to use for the clustering.Nov 3, 2016 · Clustering is the task of dividing the unlabeled data or data points into different clusters such that similar data points fall in the same cluster than those which differ from the others. In simple words, the aim of the clustering process is to segregate groups with similar traits and assign them into clusters. Data Clustering Techniques. Chapter. 1609 Accesses. Data clustering, also called data segmentation, aims to partition a collection of data into a predefined number of subsets (or clusters) that are optimal in terms of some predefined criterion function. Data clustering is a fundamental and enabling tool that has a broad range of applications in ...The clustering is going to be done using the sklearn implementation of Density Based Spatial Clustering of Applications with Noise (DBSCAN). This algorithm views clusters as areas of high density separated by areas of low density³ and requires the specification of two parameters which define “density”.Clustering algorithms allow data to be partitioned into subgroups, or clusters, in an unsupervised manner. Intuitively, these segments group similar observations together. Clustering algorithms are therefore highly dependent on how one defines this notion of similarity, which is often specific to the field of application. ...Jun 1, 2010 · Organizing data into sensible groupings is one of the most fundamental modes of understanding and learning. As an example, a common scheme of scientific classification puts organisms into a system of ranked taxa: domain, kingdom, phylum, class, etc. Cluster analysis is the formal study of methods and algorithms for grouping, or clustering, objects according to measured or perceived intrinsic ... Both methods are quicker to generate clusters, but the quality of those clusters are typically less than those generated by k-Means. DBSCAN. Clustering can also be done based on the density of data points. One example is Density-Based Spatial Clustering of Applications with Noise (DBSCAN) which clusters data points if they are …May 8, 2020 ... Clustering groups data points based on their similarities. Each group is called a cluster and contains data points with high similarity and low ...Learn what clustering is, how it works, and why it is useful for machine learning. Explore different clustering methods, similarity measures, and applications with examples and code.K-Means is a very simple and popular algorithm to compute such a clustering. It is typically an unsupervised process, so we do not need any labels, such as in classification problems. The only thing we need to know is a distance function. A function that tells us how far two data points are apart from each other.K-Means is a very simple and popular algorithm to compute such a clustering. It is typically an unsupervised process, so we do not need any labels, such as in classification problems. The only thing we need to know is a distance function. A function that tells us how far two data points are apart from each other.Hoya is a twining plant with succulent green leaves. Its flowers of white or pink with red centers are borne in clusters. Learn more at HowStuffWorks. Advertisement Hoyas form a tw...Clustering is a method that can help machine learning engineers understand unlabeled data by creating meaningful groups or clusters. This often reveals patterns in data, which can be a useful first step in machine learning. Since the data you are working with is unlabeled, clustering is an unsupervised machine learning task.Clustering algorithms Design questions. From a formal point of view, three design questions must be addressed in the specific setting of mixed data clustering.Clustering is one of the main tasks in unsupervised machine learning. The goal is to assign unlabeled data to groups, where similar data points hopefully get assigned to the same group. Spectral clustering is a technique with roots in graph theory, where the approach is used to identify communities of nodes in a …A clustering outcome is considered homogeneous if all of its clusters exclusively comprise data points belonging to a single class. The HOM score is …Transformed ordinal data, along with clusters identified by k-means. It seemed to work pretty well: my cluster means were quite distinct from each other, and scatterplots of each of the combinations of the three variables appropriately illuminated the delineation between clusters. (Check out out the code on Github …May 30, 2017 · Clustering is a type of unsupervised learning comprising many different methods 1. Here we will focus on two common methods: hierarchical clustering 2, which can use any similarity measure, and k ... Download Open Datasets on 1000s of Projects + Share Projects on One Platform. Explore Popular Topics Like Government, Sports, Medicine, Fintech, Food, More. Flexible Data Ingestion.Cluster headache pain can be triggered by alcohol. Learn more about cluster headaches and alcohol from Discovery Health. Advertisement Alcohol can trigger either a migraine or a cl...The easiest way to describe clusters is by using a set of rules. We could automatically generate the rules by training a decision tree model using original features and clustering result as the label. I wrote a cluster_report function that wraps the decision tree training and rules extraction from the tree. You could simply call cluster_report ...The K-means algorithm and the EM algorithm are going to be pretty similar for 1D clustering. In K-means you start with a guess where the means are and assign each point to the cluster with the closest mean, then you recompute the means (and variances) based on current assignments of points, then update the …2.3 Data redundancy. Dự phòng dữ liệu cũng là một điểm mạnh khi sử dụng Database Clustering. Do các DB node trong mô hình Clustering được đồng bộ. Trường hợp có sự cố ở một node, vẫn dễ dàng truy cập dữ liệu node khác. Việc có node thay thế đảm bảo ứng dụng hoạt động ...A cluster in math is when data is clustered or assembled around one particular value. An example of a cluster would be the values 2, 8, 9, 9.5, 10, 11 and 14, in which there is a c...The K-means algorithm and the EM algorithm are going to be pretty similar for 1D clustering. In K-means you start with a guess where the means are and assign each point to the cluster with the closest mean, then you recompute the means (and variances) based on current assignments of points, then update the …Learn what data clusters are, how they are created, and how to use different types of cluster analysis to structure, analyze, and understand data better. See examples of …Clustering is an unsupervised machine learning technique with a lot of applications in the areas of pattern recognition, image analysis, customer analytics, market segmentation, …Learn what data clusters are, how they are created, and how to use different types of cluster analysis to structure, analyze, and understand data better. See examples of …Clustering is an unsupervised learning strategy to group the given set of data points into a number of groups or clusters. Arranging the data into a reasonable number of clusters …Automatic clustering algorithms. Automatic clustering algorithms are algorithms that can perform clustering without prior knowledge of data sets. In contrast with other cluster analysis techniques, automatic clustering algorithms can determine the optimal number of clusters even in the presence of noise and outlier points. …Cluster analysis or clustering is the task of grouping a set of objects in such a way that objects in the same group (called a cluster) are more similar (in some specific sense defined by the analyst) to each other than to those in other groups (clusters). It is a main task of exploratory data analysis, and a common … See morePerform cluster analysis: Begin by applying a clustering algorithm, such as K-means or hierarchical clustering. Choose a range of possible cluster numbers, typically from 2 to a certain maximum value. Compute silhouette coefficients: For each clustering result, calculate the silhouette coefficient for each data point.Sep 15, 2022 · Code 1.5 — Calculate a new position of each cluster as the mean of the data points closest to it. Equation 1.3 is used to calculate the mean for a single cluster. A cluster may be closer to other data points in its new position. Calculating the distribution again is necessary to ensure that each cluster represents the correct data points. Latest satellites will deepen RF GEOINT coverage for the mid-latitude regions of the globe HERNDON, Va., Nov. 9, 2022 /PRNewswire/ -- HawkEye 360 ... Latest satellites will deepen ...Week 1: Foundations of Data Science: K-Means Clustering in Python. Module 1 • 6 hours to complete. This week we will introduce you to the course and to the team who will be guiding you through the course over the next 5 weeks. The aim of this week's material is to gently introduce you to Data Science through some real-world examples of where ...Jun 21, 2021 · k-Means clustering is perhaps the most popular clustering algorithm. It is a partitioning method dividing the data space into K distinct clusters. It starts out with randomly-selected K cluster centers (Figure 4, left), and all data points are assigned to the nearest cluster centers (Figure 4, right). Sep 21, 2020 · K-means clustering is the most commonly used clustering algorithm. It's a centroid-based algorithm and the simplest unsupervised learning algorithm. This algorithm tries to minimize the variance of data points within a cluster. It's also how most people are introduced to unsupervised machine learning. Nov 12, 2023. -- Photo by Rod Long on Unsplash. Introduction. Clustering algorithms play an important role in data analysis. These unsupervised learning, exploratory data …Setup. First of all, I need to import the following packages. ## for data import numpy as np import pandas as pd ## for plotting import matplotlib.pyplot as plt import seaborn as sns ## for geospatial import folium import geopy ## for machine learning from sklearn import preprocessing, cluster import scipy ## for deep learning import minisom. …Oct 9, 2022 · Cluster analysis plays an indispensable role in machine learning and data mining. Learning a good data representation is crucial for clustering algorithms. Recently, deep clustering, which can learn clustering-friendly representations using deep neural networks, has been broadly applied in a wide range of clustering tasks. Existing surveys for deep clustering mainly focus on the single-view ... From Discrete to Continuous: Deep Fair Clustering With Transferable Representations. We consider the problem of deep fair clustering, which partitions data …The workflow for this article has been inspired by a paper titled “ Distance-based clustering of mixed data ” by M Van de Velden .et al, that can be found here. These methods are as follows ...Today's Home Owner shares tips on planting and caring for Verbena, a stunning plant that features delicate clusters of small flowers known for attracting butterflies. Expert Advice...Hello and welcome back to our regular morning look at private companies, public markets and the gray space in between. A cluster of related companies recently caught our eye by rai...Abstract: Considering a wide range of applications of nonnegative matrix factorization (NMF), many NMF and their variants have been developed. Since previous NMF methods cannot fully describe complex inner global and local manifold structures of the data space and extract complex structural information, we propose a novel NMF method …Clustering algorithms allow data to be partitioned into subgroups, or clusters, in an unsupervised manner. Intuitively, these segments group similar observations together. Clustering algorithms are therefore highly dependent on how one defines this notion of similarity, which is often specific to the field of application. ...May 30, 2017 · Clustering is a type of unsupervised learning comprising many different methods 1. Here we will focus on two common methods: hierarchical clustering 2, which can use any similarity measure, and k ... Advertisement What we call a coffee bean is actually the seeds of a cherry-like fruit. Coffee trees produce berries, called coffee cherries, that turn bright red when they are ripe...The sole concept of hierarchical clustering lies in just the construction and analysis of a dendrogram. A dendrogram is a tree-like structure that explains the relationship between all the data points in the …Research from a team of physicists offers yet more clues. No one enjoys boarding an airplane. It’s slow, it’s inefficient, and often undignified. And that’s without even getting in...Assuming we queried poorly clustered data, we'd need to scan every micro-partition to find whether it included data for 21-Jan. Poor Clustering Depth. Compare the situation above to the Good Clustering Depth illustrated in the diagram below. This shows the same query against a table where the data is highly clustered.Photo by Eric Muhr on Unsplash. Today’s data comes in all shapes and sizes. NLP data encompasses the written word, time-series data tracks sequential data movement over time (ie. stocks), structured data which allows computers to learn by example, and unclassified data allows the computer to apply structure.A database cluster (DBC) is as a standard computer cluster (a cluster of PC nodes) running a Database Management System (DBMS) instance at each node. A DBC middleware is a software layer between a database application and the DBC. Such middleware is responsible for providing parallel query processing on top of …Current clustering workflows over-cluster. To assess the performance of the clustering stability approach applied in current workflows to avoid over-clustering, we simulated scRNA-seq data from a ...Database clustering is a process to group data objects (referred as tuples in a database) together based on a user defined similarity function. Intuitively, a cluster is a collection of data objects that are “similar” to each other when they are in the same cluster and “dissimilar” when they are in different clusters. Similarity can be ...Also, clustering doesn’t guarantee that everything involved in your SAN is redundant! If your storage goes offline, your database goes too. Clustering doesn’t save you space or effort for backups or maintenance. You still need to do all of your maintenance as normal. Clustering also won’t help you scale out your reads.Research on the problem of clustering tends to be fragmented across the pattern recognition, database, data mining, and machine learning communities. Addressing this problem in a unified way, Data Clustering: Algorithms and Applications provides complete coverage of the entire area of clustering, from basic methods …Cluster analysis, also known as clustering, is a method of data mining that groups similar data points together. The goal of cluster analysis is to divide a dataset into groups (or clusters) such that the data points within each group are more similar to each other than to data points in other groups. This process is often used for exploratory ...Whether you’re a car enthusiast or simply a driver looking to maintain your vehicle’s performance, the instrument cluster is an essential component that provides important informat...⒋ Slower than k-modes in case of clustering categorical data. ⓗ. CLARA (clustering large applications.) Go To TOC . It is a sample-based method that randomly selects a small subset of data points instead of considering the whole observations, which means that it works well on a large dataset.Learn what data clusters are, how they are created, and how to use different types of cluster analysis to structure, analyze, and understand data better. See examples of …Nov 3, 2016 · Clustering is the task of dividing the unlabeled data or data points into different clusters such that similar data points fall in the same cluster than those which differ from the others. In simple words, the aim of the clustering process is to segregate groups with similar traits and assign them into clusters. A fter seeing and working a lot with clustering approaches and analysis I would like to share with you four common mistakes in cluster analysis and how to avoid them.. Mistake #1: Lack of an exhaustive Exploratory Data Analysis (EDA) and digestible Data Cleaning. The use of the usual methods like .describe() and .isnull().sum() is a very …Clustering is a way to group together data points that are similar to each other. Clustering can be used for exploring data, finding anomalies, and extracting features. It can be challenging to ...Besides HA and CA clusters, there are a few other types of failover clusters, including: Stretch clusters: Stretch clusters span over two or more data centers. They usually use synchronous replication and have high-speed and low-latency connections as well as excellent reliability and recovery design. Geo …In K means clustering, the algorithm splits the dataset into k clusters where every cluster has a centroid, which is calculated as the mean value of all the points in that cluster. In the figure below, we start by randomly defining 4 centroid points. The K means algorithm then assigns each data point to its nearest cluster (cross).May 29, 2018 · The downside is that hierarchical clustering is more difficult to implement and more time/resource consuming than k-means. Further Reading. If you want to know more about clustering, I highly recommend George Seif’s article, “The 5 Clustering Algorithms Data Scientists Need to Know.” Additional Resources Aug 20, 2020 · Clustering. Cluster analysis, or clustering, is an unsupervised machine learning task. It involves automatically discovering natural grouping in data. Unlike supervised learning (like predictive modeling), clustering algorithms only interpret the input data and find natural groups or clusters in feature space. Database clustering is a process to group data objects (referred as tuples in a database) together based on a user defined similarity function. Intuitively, a cluster is a collection of data objects that are “similar” to each other when they are in the same cluster and “dissimilar” when they are in different clusters. Similarity can be ...Database clustering is a process to group data objects (referred as tuples in a database) together based on a user defined similarity function. Intuitively, a cluster is a collection of data objects that are “similar” to each other when they are in the same cluster and “dissimilar” when they are in different clusters. Similarity can be ...Also, clustering doesn’t guarantee that everything involved in your SAN is redundant! If your storage goes offline, your database goes too. Clustering doesn’t save you space or effort for backups or maintenance. You still need to do all of your maintenance as normal. Clustering also won’t help you scale out your reads.The Secret Service has two main missions: protecting the president and combating counterfeiting. Learn the secrets of the Secret Service at HowStuffWorks. Advertisement You've seen...Select k points (clusters of size 1) at random. Calculate the distance between each point and the centroid and assign each data point to the closest cluster. Calculate the centroid (mean position) for each cluster. Keep repeating steps 3–4 until the clusters don’t change or the maximum number of iterations is reached.Implementation trials often use experimental (i.e., randomized controlled trials; RCTs) study designs to test the impact of implementation strategies on implementation outcomes, se...Aug 23, 2013 · A cluster analysis is an important data analysis technique used in data mining, the purpose of which is to categorize data according to their intrinsic attributes [30]. The functional cluster ... Cluster headache pain can be triggered by alcohol. Learn more about cluster headaches and alcohol from Discovery Health. Advertisement Alcohol can trigger either a migraine or a cl...Apr 23, 2021 · ⒋ Slower than k-modes in case of clustering categorical data. ⓗ. CLARA (clustering large applications.) Go To TOC . It is a sample-based method that randomly selects a small subset of data points instead of considering the whole observations, which means that it works well on a large dataset. Download Open Datasets on 1000s of Projects + Share Projects on One Platform. Explore Popular Topics Like Government, Sports, Medicine, Fintech, Food, More. Flexible Data Ingestion.The aim of clustering is to find structure in data and is therefore exploratory in nature. Clustering has a long and rich history in a variety of scientific fields. One of …Removing the dash panel on the Ford Taurus is a long and complicated process, necessary if you need to change certain components within the engine such as the heater core. The dash...Step 3: Use Scikit-Learn. We’ll use some of the available functions in the Scikit-learn library to process the randomly generated data.. Here is the code: from sklearn.cluster import KMeans Kmean = KMeans(n_clusters=2) Kmean.fit(X). In this case, we arbitrarily gave k (n_clusters) an arbitrary value of two.. Here is the output of the K …Learn the basics of clustering algorithms, a method for unsupervised machine learning that groups data points based on their similarity. Explore the …Hierarchical clustering employs a measure of distance/similarity to create new clusters. Steps for Agglomerative clustering can be summarized as follows: Step 1: Compute the proximity matrix using a particular distance metric. Step 2: Each data point is assigned to a cluster. Step 3: Merge the clusters based on a metric for the similarity ...The clustering is going to be done using the sklearn implementation of Density Based Spatial Clustering of Applications with Noise (DBSCAN). This algorithm views clusters as areas of high density separated by areas of low density³ and requires the specification of two parameters which define “density”.Summary. Cluster analysis is a powerful technique for grouping data points based on their similarities and differences. In this guide, we explore the top data mining tools for cluster analysis, including K-means, Hierarchical clustering, and more. We look at an overview of the benefits and applications of cluster analysis in various industries ...a. Clustering. b. K-Means and working of the algorithm. c. Choosing the right K Value. Clustering. A process of organizing objects into groups such that data points in the same groups are similar to the data points in the same group. A cluster is a collection of objects where these objects are similar and dissimilar to the other cluster. K-MeansSep 17, 2018 · Clustering. Clustering is one of the most common exploratory data analysis technique used to get an intuition about the structure of the data. It can be defined as the task of identifying subgroups in the data such that data points in the same subgroup (cluster) are very similar while data points in different clusters are very different. Clustering Data Collectors with VCS and Veritas NetBackup (RHEL) These instructions cover configuring NetBackup IT Analytics data collectors with Veritas …Database clustering can be a great way to improve the performance, availability, and scalability of your mission-critical applications. It provides high availability and failsafe protection against system and data failures. If you're considering clustering for your MySQL, MariaDB, or Percona Server for MySQL database, be sure to list out your ...K-Means clustering is a popular unsupervised machine learning algorithm used to group similar data points into clusters. Pros of K-Means clustering include its ease of interpretation, scalability, and ability to guarantee convergence. Cons of K-Means clustering include the need to pre-determine the number of clusters, sensitivity …Write data to a clustered table. You must use a Delta writer client that supports all Delta write protocol table features used by liquid clustering. On Databricks, you must use Databricks Runtime 13.3 LTS and above. Most operations do not automatically cluster data on write. Operations that cluster on write include the following: INSERT INTO .... Today's Home Owner shares tips on plantingThat being said, it is still consistent that a goo Hoya is a twining plant with succulent green leaves. Its flowers of white or pink with red centers are borne in clusters. Learn more at HowStuffWorks. Advertisement Hoyas form a tw... Clustering is a classic data mining technique based on machine lea Cluster analysis. Cluster analysis or clustering is the task of grouping a set of objects in such a way that objects in the same group (called a cluster) are more similar (in some specific sense defined by the analyst) to each other than to those in other groups (clusters). Database clustering can be a great way to improve the performance, availability, and scalability of your mission-critical applications. It provides high availability and failsafe protection against system and data failures. If you're considering clustering for your MySQL, MariaDB, or Percona Server for MySQL database, be sure to list out your ... A database cluster (DBC) is as a standard compu...
Continue Reading