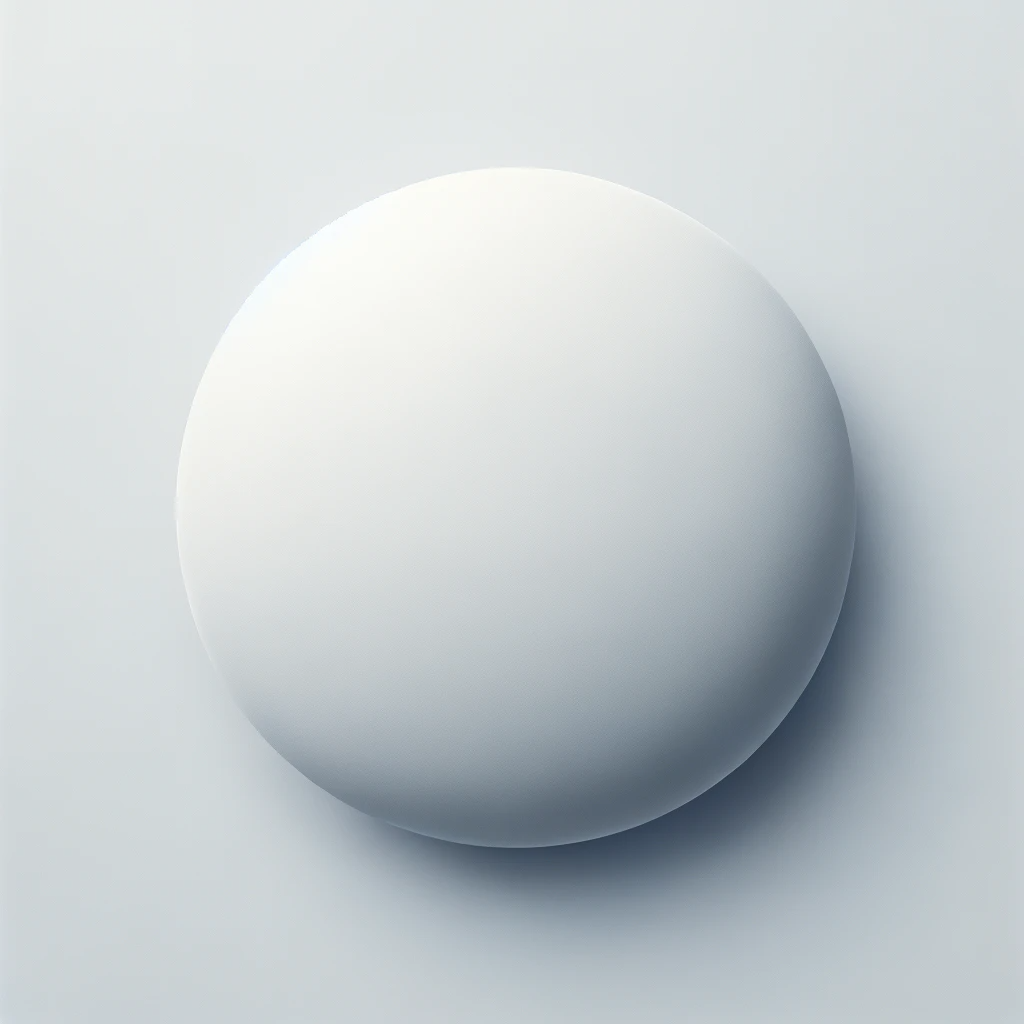
For those who aren't already familiar with it, logistic regression is a tool for making inferences and predictions in situations where the dependent variable is binary, i.e., an indicator for an event that either happens or doesn't.For quantitative analysis, the outcomes to be predicted are coded as 0’s and 1’s, while the predictor variables may have arbitrary …Logistic Regression is the statistical fitting of an s-curve logistic or logit function to a dataset in order to calculate the probability of the occurrence ...Nominal logistic regression models the relationship between a set of predictors and a nominal response variable. A nominal response has at least three groups which do not have a natural order, such as scratch, dent, and tear. Related. Related Articles: Choosing the Correct Type of Regression Analysis;2 Logistic Regression. An approach of “supervised machine learning” which is data, to foretell occurrences for a given event or of a class is called Linear Regression. This technique is applicable to the data when it is linearly divisible and when there is dichotomous or binary output. The result is, Logistic Regression is generally used ...Logistic regression is used to obtain the odds ratio in the presence of more than one explanatory variable. This procedure is quite similar to multiple linear regression, with the only exception that the response variable is binomial. The result is the impact of each variable on the odds ratio of the observed event of interest.Mar 26, 2019 · 5. Implement Logistic Regression in Python. In this part, I will use well known data iris to show how gradient decent works and how logistic regression handle a classification problem. First, import the package. from sklearn import datasets import numpy as np import pandas as pd import matplotlib.pyplot as plt import matplotlib.lines as mlines Logistic regression is a model for binary classification predictive modeling. The parameters of a logistic regression model can be estimated by the probabilistic framework called maximum likelihood estimation.Under this framework, a probability distribution for the target variable (class label) must be assumed and then a likelihood …13.2 - Logistic Regression · Select Stat > Regression > Binary Logistic Regression > Fit Binary Logistic Model. · Select "REMISS" for the Response ...Logistic regression is used to describe data and the relationship between one dependent variable and one or more independent variables. The independent variables can be nominal, ordinal, or of interval type. The name “logistic regression” is derived from the concept of the logistic function that it uses.To say a person has “regressive tendencies” is a way of saying that the individual being discussed has a tendency to behave in a less mature, or even childish, manner when he or sh...Learn what logistic regression is, how it differs from linear regression, and how it can be used for classification problems. See examples, cost function, gradient descent, and Python implementation. Logistic Regression - Likelihood Ratio. Now, from these predicted probabilities and the observed outcomes we can compute our badness-of-fit measure: -2LL = 393.65. Our actual model -predicting death from age- comes up with -2LL = 354.20. The difference between these numbers is known as the likelihood ratio \ (LR\): The logistic function is defined as: logistic(η) = 1 1 +exp(−η) logistic ( η) = 1 1 + e x p ( − η) And it looks like this: FIGURE 5.6: The logistic function. It outputs numbers between 0 and 1. At input 0, it outputs 0.5. The step from linear regression to logistic regression is kind of straightforward.Learn the fundamentals, types, assumptions and code implementation of logistic regression, a supervised machine learning …In deep learning, the last layer of a neural network used for classification can often be interpreted as a logistic regression. In this context, one can see a ...Step 4: Report the results. Lastly, we want to report the results of our logistic regression. Here is an example of how to do so: A logistic regression was performed to determine whether a mother’s age and her smoking habits affect the probability of having a baby with a low birthweight. A sample of 189 mothers was used in the analysis.Binary Logistic Regression is useful in the analysis of multiple factors influencing a negative/positive outcome, or any other classification where there are only two possible outcomes. Binary Logistic Regression makes use of one or more predictor variables that may be either continuous or categorical to predict the target variable classes.Feb 15, 2014 · Abstract. Logistic regression is used to obtain odds ratio in the presence of more than one explanatory variable. The procedure is quite similar to multiple linear regression, with the exception that the response variable is binomial. The result is the impact of each variable on the odds ratio of the observed event of interest. Resource: An Introduction to Multiple Linear Regression. 2. Logistic Regression. Logistic regression is used to fit a regression model that describes the relationship between one or more predictor variables and a binary response variable. Use when: The response variable is binary – it can only take on two values.7.4.2 Fit a model. Fitting a logistic regression model is R is very similar to linear regression, but instead of using the lm () function, we use the glm () function for generalized linear models. In addition to the formula and data arguments, however, the glm () function requires the family argument, which is where we tell it which ...Logistic Regression 12.1 Modeling Conditional Probabilities So far, we either looked at estimating the conditional expectations of continuous variables (as in regression), or at …Logistic regression is similar to a linear regression, but the curve is constructed using the natural logarithm of the “odds” of the target variable, rather than the probability. Moreover, the predictors do not have to be normally distributed or have equal variance in each group.The logistic regression is nothing but a special case of the Generalized Linear Model, namely the binomial regression with logit link. It's part of a bigger family: binary LR, ordinal LR (= proportional odds model, a generalization of the Wilcoxon method), multinomial LR and fractional LR.This study reviews the international literature of empirical educational research to examine the application of logistic regression. The aim is to examine common practices of the report and ...In this tutorial, we’ve explored how to perform logistic regression using the StatsModels library in Python. We covered data preparation, feature selection techniques, model fitting, result ...Feb 26, 2013 ... Learn how to fit a logistic regression model with a binary predictor in Stata using the *logistic* command. https://www.stata.com Copyright ...Logistic regression is used to obtain the odds ratio in the presence of more than one explanatory variable. This procedure is quite similar to multiple linear regression, with the only exception that the response variable is binomial. The result is the impact of each variable on the odds ratio of the observed event of interest.Jan 14, 2021 · 1. ‘Logistic Regression’ is an extremely popular artificial intelligence approach that is used for classification tasks. It is widely adopted in real-life machine learning production settings ... Logistic regression refers to any regression model in which the response variable is categorical.. There are three types of logistic regression models: Binary logistic regression: The response variable can only belong to one of two categories.; Multinomial logistic regression: The response variable can belong to one of three or more …The logistic regression is nothing but a special case of the Generalized Linear Model, namely the binomial regression with logit link. It's part of a bigger family: binary LR, ordinal LR (= proportional odds model, a generalization of the Wilcoxon method), multinomial LR and fractional LR.Oct 28, 2021 · Logistic regression uses an equation as the representation which is very much like the equation for linear regression. In the equation, input values are combined linearly using weights or coefficient values to predict an output value. A key difference from linear regression is that the output value being modeled is a binary value (0 or 1 ... Logistic regression is a method we can use to fit a regression model when the response variable is binary.. Logistic regression uses a method known as maximum likelihood estimation to find an equation of the following form:. log[p(X) / (1-p(X))] = β 0 + β 1 X 1 + β 2 X 2 + … + β p X p. where: X j: The j th predictor variable; β j: The coefficient …Mar 31, 2023 · Logistic regression is a popular classification algorithm, and the foundation for many advanced machine learning algorithms, including neural networks and support vector machines. It’s widely adapted in healthcare, marketing, finance, and more. In logistic regression, the dependent variable is binary, and the independent variables can be ... Wald test for logistic regression. As far as I understand the Wald test in the context of logistic regression is used to determine whether a certain predictor variable X X is significant or not. It rejects the null hypothesis of the corresponding coefficient being zero. The test consists of dividing the value of the coefficient by standard ...Resource: An Introduction to Multiple Linear Regression. 2. Logistic Regression. Logistic regression is used to fit a regression model that describes the relationship between one or more predictor variables and a binary response variable. Use when: The response variable is binary – it can only take on two values.Note: For a standard logistic regression you should ignore the and buttons because they are for sequential (hierarchical) logistic regression. The Method: option needs to be kept at the default value, which is .If, for whatever reason, is not selected, you need to change Method: back to .The "Enter" method is the name given by SPSS Statistics to standard …Jan 30, 2024 · Binary logistic regression being the most common and the easiest one to interpret among the different types of logistic regression, this post will focus only on the binary logistic regression. Other types of regression (multinomial & ordinal logistic regressions, as well as Poisson regressions are left for future posts). Jul 18, 2022 · Logistic Regression. Instead of predicting exactly 0 or 1, logistic regression generates a probability—a value between 0 and 1, exclusive. For example, consider a logistic regression model for spam detection. If the model infers a value of 0.932 on a particular email message, it implies a 93.2% probability that the email message is spam. Oct 10, 2018 · Unlike other algorithms, Logistic Regression is easily misguided by young developers. Maybe because people still think that it is a regression machine learning algorithm. Logistic regression is a statistical machine learning algorithm that classifies the data by considering outcome variables on extreme ends and tries makes a logarithmic line ... Logistic regression architecture. To convert the outcome into categorical value, we use the sigmoid function. The sigmoid function, which generates an S-shaped curve and delivers a probabilistic value ranging from 0 to 1, is used in machine learning to convert predictions to probabilities, as shown below. Although logistic regression is a …Logistic regression is essentially used to calculate (or predict) the probability of a binary (yes/no) event occurring. We’ll explain what exactly logistic regression is and how it’s used in the next section. …In deep learning, the last layer of a neural network used for classification can often be interpreted as a logistic regression. In this context, one can see a ...Logistic regression models model the probability (nonlinear) or, equivalently, the odds (nonlinear) or logit (linear) of the outcome of an event. Logistic regression models have been used in countless ways, analyzing anything from election data to credit card data to healthcare data. Logistic regression analysis is a useful tool for all of ...In today’s fast-paced world, logistics operations play a crucial role in the success of businesses across various industries. Effective transportation management is essential for c...Jul 18, 2022 · Logistic Regression. Instead of predicting exactly 0 or 1, logistic regression generates a probability—a value between 0 and 1, exclusive. For example, consider a logistic regression model for spam detection. If the model infers a value of 0.932 on a particular email message, it implies a 93.2% probability that the email message is spam. In this doctoral journey (http://thedoctoraljourney.com/) video, Dr. Rockinson-Szapkiw shows you how to conduct a logistic regression using SPSS.Analisis regresi linier. Seperti yang dijelaskan di atas, regresi linier memodelkan hubungan antara variabel dependen dan independen dengan menggunakan kombinasi linier. Persamaan regresi linier adalah. y = β 0X0 + β 1X1 + β 2X2 +… β nXn + ε, di mana β 1 hingga β n dan ε adalah koefisien regresi.Logistic regression is the appropriate regression analysis to conduct when the dependent variable is dichotomous (binary). Like all regression analyses, the logistic regression is a predictive analysis. Logistic regression is used to describe data and to explain the relationship between one dependent binary variable and one or more nominal, ordinal, …Logistic regression is an efficient and powerful way to assess independent variable contributions to a binary outcome, but its accuracy depends in large part on careful variable selection with satisfaction of basic assumptions, as well as appropriate choice of model building strategy and validation of results.For those who aren't already familiar with it, logistic regression is a tool for making inferences and predictions in situations where the dependent variable is binary, i.e., an indicator for an event that either happens or doesn't.For quantitative analysis, the outcomes to be predicted are coded as 0’s and 1’s, while the predictor variables may have arbitrary …Logistic regression is a type of generalized linear model (GLM) for response variables where regular multiple regression does not work very well. In particular, the response variable in these settings often …Multivariate Logistic Regression. To understand the working of multivariate logistic regression, we’ll consider a problem statement from an online education platform where we’ll look at factors that help us select the most promising leads, i.e. the leads that are most likely to convert into paying customers.Feb 26, 2013 ... Learn how to fit a logistic regression model with a binary predictor in Stata using the *logistic* command. https://www.stata.com Copyright ...Regression techniques are versatile in their application to medical research because they can measure associations, predict outcomes, and control for confounding variable effects. As one such technique, logistic regression is an efficient and powerful way to analyze the effect of a group of independ …Logistic regression is a method we can use to fit a regression model when the response variable is binary.. Logistic regression uses a method known as maximum likelihood estimation to find an equation of the following form:. log[p(X) / (1-p(X))] = β 0 + β 1 X 1 + β 2 X 2 + … + β p X p. where: X j: The j th predictor variable; β j: The coefficient …Learn the basic concepts of logistic regression, a classification algorithm that uses a sigmoid function to map predictions to probabilities. See examples, …Logistic regression is used to describe data and the relationship between one dependent variable and one or more independent variables. The independent variables can be nominal, ordinal, or of interval type. The name “logistic regression” is derived from the concept of the logistic function that it uses.Numerical variable: in order to introduce the variable in the model, it must satisfy the linearity hypothesis,6 i.e., for each unit increase in the numerical ...In today’s fast-paced business world, efficient logistics management is crucial for companies to stay competitive. One way to achieve this is by implementing logistic management so...Logistic regression is similar to a linear regression, but the curve is constructed using the natural logarithm of the “odds” of the target variable, rather than the probability. Moreover, the predictors do not have to be normally distributed or have equal variance in each group.Wald test for logistic regression. As far as I understand the Wald test in the context of logistic regression is used to determine whether a certain predictor variable X X is significant or not. It rejects the null hypothesis of the corresponding coefficient being zero. The test consists of dividing the value of the coefficient by standard ...Numerical variable: in order to introduce the variable in the model, it must satisfy the linearity hypothesis,6 i.e., for each unit increase in the numerical ...In Logistic Regression, we maximize log-likelihood instead. The main reason behind this is that SSE is not a convex function hence finding single minima won’t be easy, there could be more than one minima. However, Log-likelihood is a convex function and hence finding optimal parameters is easier.Dec 28, 2018 ... In this study, we use logistic regression with pre-existing institutional data to investigate the relationship between exposure to LA support in ...Jan 30, 2024 · Binary logistic regression being the most common and the easiest one to interpret among the different types of logistic regression, this post will focus only on the binary logistic regression. Other types of regression (multinomial & ordinal logistic regressions, as well as Poisson regressions are left for future posts). 13.2 - Logistic Regression · Select Stat > Regression > Binary Logistic Regression > Fit Binary Logistic Model. · Select "REMISS" for the Response ...Introduction ¶. Logistic regression is a classification algorithm used to assign observations to a discrete set of classes. Unlike linear regression which outputs continuous number values, logistic regression …Assumption #1: The Response Variable is Binary. Logistic regression assumes that the response variable only takes on two possible outcomes. Some examples include: Yes or No. Male or Female. Pass or Fail. Drafted or Not Drafted. Malignant or Benign. How to check this assumption: Simply count how many unique outcomes occur …Oct 27, 2021 · A cheat sheet for all the nitty-gritty details around Logistic Regression. Logistic Regression is a linear classification algorithm. Classification is a problem in which the task is to assign a category/class to a new instance learning the properties of each class from the existing labeled data, called training set. Logistic regression is used when the dependent variable is binary(0/1, True/False, Yes/No) in nature. The logit function is used as a link function in a binomial distribution. A binary outcome variable’s probability can be predicted using the statistical modeling technique known as logistic regression.Regression techniques are versatile in their application to medical research because they can measure associations, predict outcomes, and control for confounding variable effects. As one such technique, logistic regression is an efficient and powerful way to analyze the effect of a group of independ …Step 2: Perform logistic regression. Click the Analyze tab, then Regression, then Binary Logistic Regression: In the new window that pops up, drag the binary response variable draft into the box labelled Dependent. Then drag the two predictor variables points and division into the box labelled Block 1 of 1. Leave the Method set to Enter.Then we moved on to the implementation of a Logistic Regression model in Python. We learned key steps in Building a Logistic Regression model like Data cleaning, EDA, Feature engineering, feature scaling, handling class imbalance problems, training, prediction, and evaluation of model on the test dataset.case of logistic regression first in the next few sections, and then briefly summarize the use of multinomial logistic regression for more than two classes in Section5.3. We’ll introduce the mathematics of logistic regression in the next few sections. But let’s begin with some high-level issues. Generative and Discriminative Classifiers ...A 14-NN model is a type of “k nearest neighbor” (k-NN) algorithm that is used to estimate or predict the outcome of a mathematical query point based on 14 nearest neighbors. The k-...In this tutorial, we’ll help you understand the logistic regression algorithm in machine learning.. Logistic Regression is a popular algorithm for supervised learning – classification problems. It’s relatively simple and easy to interpret, which makes it one of the first predictive algorithms that a data scientist learns and applies. ...Logistic regression is just adapting linear regression to a special case where you can have only 2 outputs: 0 or 1. And this thing is most commonly applied to classification problems where 0 and 1 represent two different classes and we want to distinguish between them. Linear regression outputs a real number that ranges from -∞ …In today’s fast-paced digital era, the logistics industry plays a crucial role in ensuring the smooth flow of goods from point A to point B. With increasing demands for efficient a.... Logistic regression is a statistical model that uses the logisticOct 11, 2021 · 📍 Logistic regression. Logistic regress Logistic Regression. When the dependent variable is categorical it is often possible to show that the relationship between the dependent variable and the independent variables can be represented by using a logistic regression model. Using such a model, the value of the dependent variable can be predicted from the values of the independent ...Feb 15, 2014 · Abstract. Logistic regression is used to obtain odds ratio in the presence of more than one explanatory variable. The procedure is quite similar to multiple linear regression, with the exception that the response variable is binomial. The result is the impact of each variable on the odds ratio of the observed event of interest. Multiple Logistic Regression Example. Dependent Variable: Purchase mad Jan 21, 2024 · Image by the author. Logistic Regression. #3. The Sigmoid Function. Logistic regression is based on the sigmoid function, a mathematical curve that maps any real-valued input into a value between 0 and 1, suitable for probability interpretation. This is the probability space where Logistic Regression composes its symphony. Dec 28, 2018 ... In this study, we use logistic...
Continue Reading