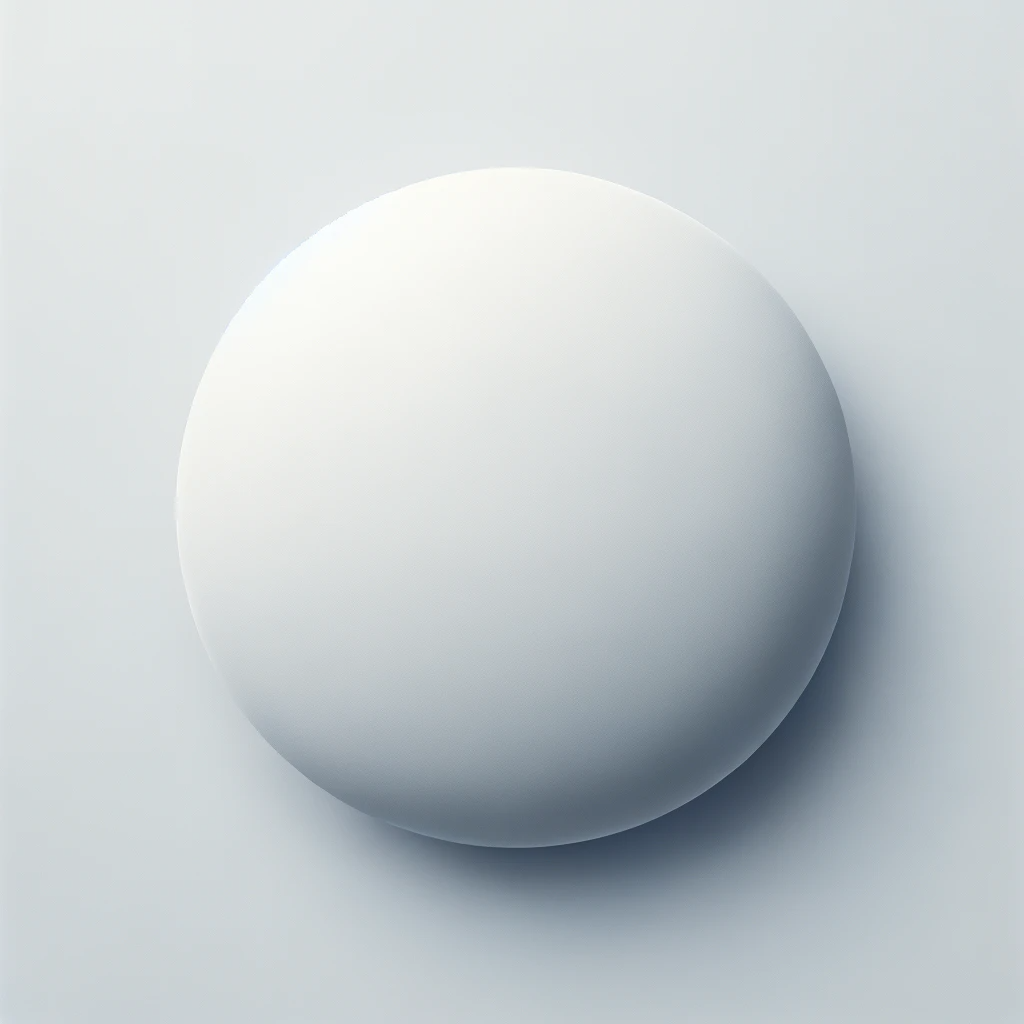
Clustering is an unsupervised learning method having models – KMeans, hierarchical clustering, DBSCAN, etc. Visual representation of clusters shows the data in an easily understandable format as it groups elements of a large dataset according to their similarities. This makes analysis easy.Joint clustering and feature learning methods have shown remarkable performance in unsupervised representation learning. However, the training schedule alternating between feature clustering and network parameters update leads to unstable learning of visual representations. To overcome this challenge, we propose Online Deep …Hello and welcome back to our regular morning look at private companies, public markets and the gray space in between. A cluster of related companies recently caught our eye by rai...Whether you’re a car enthusiast or simply a driver looking to maintain your vehicle’s performance, the instrument cluster is an essential component that provides important informat...I have an unsupervised K-Means clustering model output (as shown in the first photo below) and then I clustered my data using the actual classifications. The photo below are the actual classifications. I am trying to test, in Python, how well my K-Means classification (above) did against the actual classification. ...Unsupervised clustering is of central importance for the analysis of the scRNA-seq data, as it can be used to identify putative cell types. However, due to noise impacts, high dimensionality and pervasive dropout events, clustering analysis of scRNA-seq data remains a computational challenge.K-Means clustering is an unsupervised machine learning algorithm that is used to solve clustering problems. The goal of this algorithm is to find groups or clusters in the data, …Jan 1, 2021 · The commonly used unsupervised learning technique is cluster analysis, which is massively utilized for exploratory data analysis to determine the hidden patterns and to group the data. Here, a review of unsupervised learning techniques is done for performing data clustering on massive datasets. Given that dealing with unlabelled data is one of the main use cases of unsupervised learning, we require some other metrics that evaluate clustering results without needing to refer to ‘true’ labels. Suppose we have the following results from three separate clustering analyses. Evidently, the ‘tighter’ we can make our clusters, the better.K-means is one of the simplest unsupervised learning algorithms that solve the well known clustering problem. The procedure follows a simple and easy way to classify a given data set through a certain number of clusters (assume k clusters) fixed a priori. The main idea is to define k centroids, one for each cluster.Our approach therefore preserves the structure of a deep scattering network while learning a representation relevant for clustering. It is an unsupervised representation learning method located in ...To overcome the shortcomings of the existing approaches, we introduce a new algorithm for key frame extraction based on unsupervised clustering. The proposed algorithm is both computationally simple and able to adapt to the visual content. The efficiency and effectiveness are validated by large amount of real-world videos. ...What is Clustering? “Clustering” is the process of grouping similar entities together. The goal of this unsupervised machine learning technique is to find similarities …Unsupervised machine learning, and in particular data clustering, is a powerful approach for the analysis of datasets and identification of characteristic features …Unsupervised image clustering. The primary purpose of UIC is to assign similar images to the same group. Since DNNs achieve superior performance for machine vision tasks [22], deep image clustering approaches tend to utilize DNNs to perform this task. However, the similarity of visual features across different semantic classes often …GibbsCluster is a powerful tool for unsupervised motif discovery because it can simultaneously cluster and align peptide data. The GibbsCluster 2.0 presented here is an improved version incorporating insertion and deletions accounting for variations in motif length in the peptide input. In basic terms, the program takes as input a set of ...K-means. K-means is an unsupervised learning method for clustering data points. The algorithm iteratively divides data points into K clusters by minimizing the variance in each cluster. Here, we will show you how to estimate the best value for K using the elbow method, then use K-means clustering to group the data points into clusters.Then, an unsupervised cluster method is used to produce dense regions. Each adjusted dense region is fed into the detector for object detection. Finally, a global merge module generates the final predict results. Experiments were conducted on two popular aerial image datasets including VisDrone2019 and UAVDT. In both datasets, our proposed ...16-Aug-2014 ... Using unsupervised learning to reduce the dimensionality and then using supervised learning to obtain an accurate predictive model is commonly ...DeLUCS is the first method to use deep learning for accurate unsupervised clustering of unlabelled DNA sequences. The novel use of deep learning in this context significantly boosts the classification accuracy (as defined in the Evaluation section), compared to two other unsupervised machine learning clustering methods (K-means++ …Feb 17, 2023 · Abstract. Unsupervised clustering is useful for automated segregation of participants, grouping of entities, or cohort phenotyping. Such derived computed cluster labels may be critical for identifying similar traits, characterizing common behaviors, delineating natural boundaries, or categorizing heterogeneous objects or phenomena. Use the following steps to access unsupervised machine learning in DSS: Go to the Flow for your project. Click on the dataset you want to use. Select the Lab. Create a new visual analysis. Click on the Models tab. Select Create first model. Select AutoML Clustering.Step 3: Use Scikit-Learn. We’ll use some of the available functions in the Scikit-learn library to process the randomly generated data.. Here is the code: from sklearn.cluster import KMeans Kmean = KMeans(n_clusters=2) Kmean.fit(X). In this case, we arbitrarily gave k (n_clusters) an arbitrary value of two.. Here is the output of the K …The scABC framework for unsupervised clustering of scATAC-seq data.a Overview of scABC pipeline.scABC constructs a matrix of read counts over peaks, then weights cells by sample depth and applies ...GibbsCluster is a powerful tool for unsupervised motif discovery because it can simultaneously cluster and align peptide data. The GibbsCluster 2.0 presented here is an improved version incorporating insertion and deletions accounting for variations in motif length in the peptide input. In basic terms, the program takes as input a set of ...Word vectors can be used to construct vectors for words or sentences, to use them for similarity or clustering tasks. Even easy tasks like plotting a word cloud for a dataset is a powerful method to analyze a dataset. However, the real power of word-vectors is unleashed with Language Modelling.Unsupervised clustering models have been widely used for multimetric phenotyping of complex and heterogeneous diseases such as diabetes and obstructive sleep apnea (OSA) to more precisely ...K-means is an unsupervised clustering algorithm designed to partition unlabelled data into a certain number (thats the “ K”) of distinct groupings.In other words, k-means finds observations that share important characteristics and …09-Sept-2023 ... Unsupervised learning is critical in logistics and supply chain management for optimising delivery routes and inventory management. Clustering ...Given that dealing with unlabelled data is one of the main use cases of unsupervised learning, we require some other metrics that evaluate clustering results without needing to refer to ‘true’ labels. Suppose we have the following results from three separate clustering analyses. Evidently, the ‘tighter’ we can make our clusters, the better.This method is also mentioned in the question Evaluation measure of clustering, linked in the comments for this question. If your unsupervised learning method is probabilistic, another option is to evaluate some probability measure (log-likelihood, perplexity, etc) on held out data. The motivation here is that if your unsupervised …K-means doesn't allow noisy data, while hierarchical clustering can directly use the noisy dataset for clustering. t-SNE Clustering. One of the unsupervised learning methods for visualization is t-distributed stochastic neighbor embedding, or t-SNE. It maps high-dimensional space into a two or three-dimensional space which can then be visualized.Clustering falls under the unsupervised learning technique. In this technique, the data is not labelled and there is no defined dependant variable. ... Clustering is all about distance between two points and distance between two clusters. Distance cannot be negative. There are a few common measures of distance that the algorithm uses for …Clouds and Precipitation - Clouds and precipitation make one of the best meteorological teams. Learn why clouds and precipitation usually mean good news for life on Earth. Advertis...Clustering methods. There are three main clustering methods in unsupervised learning, namely partitioning, hierarchical and density based methods. Each method has its own strategy of separating ...Clustering is a critical step in single cell-based studies. Most existing methods support unsupervised clustering without the a priori exploitation of any domain knowledge. When confronted by the ...Then, an unsupervised cluster method is used to produce dense regions. Each adjusted dense region is fed into the detector for object detection. Finally, a global merge module generates the final predict results. Experiments were conducted on two popular aerial image datasets including VisDrone2019 and UAVDT. In both datasets, our proposed ...We present an unsupervised deep embedding algorithm, the Deep Convolutional Autoencoder-based Clustering (DCAEC) model, to cluster label-free IFC …Deep Learning (DL) has shown great promise in the unsupervised task of clustering. That said, while in classical (i.e., non-deep) clustering the benefits of the nonparametric approach are well known, most deep-clustering methods are parametric: namely, they require a predefined and fixed number of clusters, denoted by K. When K is …Clustering is an unsupervised learning exploratory technique, that allows identifying structure in the data without prior knowledge on their distribution. The main idea is to classify the objects ...Clustering and association are two of the most important types of unsupervised learning algorithms. Today, we will be focusing only on Clustering. …K-means clustering is the most commonly used clustering algorithm. It's a centroid-based algorithm and the simplest unsupervised learning algorithm. This algorithm tries to minimize the variance of data points within a cluster. It's also how most people are introduced to unsupervised machine learning.Unsupervised clustering is perhaps one of the most important tasks of unsupervised machine learning algorithms currently, due to a variety of application needs and connections with other problems. Clustering can be formulated as follows. Consider a dataset that is composed of N samples ...When it comes to vehicle repairs, finding cost-effective solutions is always a top priority for car owners. One area where significant savings can be found is in the replacement of...clustering,itdiffersfromtwokeyaspects: (1)weintroduce the Clusformer, a simple but new perspective of Trans-former based approach, to automatic unsupervised visual clustering via its efficient unsupervised attention mecha-nism. (2) our method is able to robustly deal with noisy or hard samples. It’s also flexible and effective to col-Unsupervised clustering analysis categorized the patients into two subtypes by 2483 IRGs. Our findings revealed that the OS in patients with subtype 2 exhibited a notably greater value compared to subtype 1, suggesting that these IRGs may potentially impact the prognosis of ACC. To enhance the investigation of the involvement …Something went wrong and this page crashed! If the issue persists, it's likely a problem on our side. Unexpected token < in JSON at position 4. SyntaxError: Unexpected token < in JSON at position 4. Refresh. Explore and run machine learning code with Kaggle Notebooks | Using data from 20 Newsgroup Sklearn.Earth star plants quickly form clusters of plants that remain small enough to be planted in dish gardens or terrariums. Learn more at HowStuffWorks. Advertisement Earth star plant ...May 30, 2017 · Clustering finds patterns in data—whether they are there or not. Many biological analyses involve partitioning samples or variables into clusters on the basis of similarity or its converse ... Earth star plants quickly form clusters of plants that remain small enough to be planted in dish gardens or terrariums. Learn more at HowStuffWorks. Advertisement Earth star plant ...Unsupervised Manifold Linearizing and Clustering. Tianjiao Ding, Shengbang Tong, Kwan Ho Ryan Chan, Xili Dai, Yi Ma, Benjamin D. Haeffele. We consider the problem of simultaneously clustering and learning a linear representation of data lying close to a union of low-dimensional manifolds, a fundamental task in machine learning …Unsupervised learning is a useful technique for clustering data when your data set lacks labels. Once clustered, you can further study the data set to identify hidden features of that data. This tutorial …Want to know how to make a schedule for kids after-school? Visit HowStuffWorks Family to learn how to make a schedule for kids after-school. Advertisement Gone are the days when ki...Clustering is a technique in machine learning that attempts to find groups or clusters of observations within a dataset such that th e observations within each cluster are quite similar to each other, while observations in different clusters are quite different from each other.. Clustering is a form of unsupervised learning because we’re simply …Detection and removal of outliers in a dataset is a fundamental preprocessing task without which the analysis of the data can be misleading. Furthermore, the existence of anomalies in the data can heavily degrade the performance of machine learning algorithms. In order to detect the anomalies in a dataset in an unsupervised manner, some novel …K-means clustering is the most commonly used clustering algorithm. It's a centroid-based algorithm and the simplest unsupervised learning algorithm. This algorithm tries to minimize the variance of data points within a cluster. It's also how most people are introduced to unsupervised machine learning.One of the more common goals of unsupervised learning is to cluster the data, to find reasonable groupings where the points in each group seem more similar to …Looking for an easy way to stitch together a cluster of photos you took of that great vacation scene? MagToo, a free online panorama-sharing service, offers a free online tool to c...Unsupervised landmarks discovery (ULD) for an object category is a challenging computer vision problem. In pursuit of developing a robust ULD framework, …Clustering is an unsupervised learning method having models – KMeans, hierarchical clustering, DBSCAN, etc. Visual representation of clusters shows the data in an easily understandable format as it groups elements of a large dataset according to their similarities. This makes analysis easy.CNNI uses a Neural Network to cluster data points. Training of the Neural Network mimics supervised learning, with an internal clustering evaluation index acting as the loss function. It successively adjusts the weights of the Neural Network to reduce the loss (improve the value of the index). The structure of CNNI is simple: a Neural Network ...Joint clustering and feature learning methods have shown remarkable performance in unsupervised representation learning. However, the training schedule alternating between feature clustering and network parameters update leads to unstable learning of visual representations. To overcome this challenge, we propose Online Deep …"I go around Yaba and it feels like more hype than reality compared to Silicon Valley." For the past few years, the biggest question over Yaba, the old Lagos neighborhood that has ...Clustering algorithms form groupings in such a way that data within a group (or cluster) have a higher measure of similarity than data in any other cluster. Various similarity measures can be used, including Euclidean, probabilistic, cosine distance, and correlation. Most unsupervised learning methods are a form of cluster analysis.Advertisement Deep-sky objects include multiple stars, variable stars, star clusters, nebulae and galaxies. A catalog of more than 100 deep-sky objects that you can see in a small ...Unsupervised clustering reveals clusters of learners with differing online engagement. To find groups of learners with similar online engagement in an unsupervised manner, we follow the procedure ...Example of Unsupervised Learning: K-means clustering. Let us consider the example of the Iris dataset. This is a table of data on 150 individual plants belonging to three species. For each plant, there are four measurements, and the plant is also annotated with a target, that is the species of the plant. The data can be easily represented in a ...In contrast customers in cluster 0 prefer to pay with cash the most.Cluster 0 also has the highest number of orders with 2072 orders while cluster 1 has 93 orders, being the least amount of orders out of the 5 clusters.In terms of profit cluster 0 generates the app the most amount of profit followed by cluster 3,2,1, and 4.This paper presents an autoencoder and K-means clustering-based unsupervised technique that can be used to cluster PQ events into categories like sag, interruption, transients, normal, and harmonic distortion to enable filtering of anomalous waveforms from recurring or normal waveforms. The method is demonstrated using three …Unsupervised Clustering of Southern Ocean Argo Float Temperature Profiles. Daniel C. Jones, Corresponding Author. Daniel C. Jones [email protected] ... GMM is a generalization of k-means clustering, which only attempts to minimize in-group variance by shifting the means. By contrast, GMM attempts to identify means and standard …Step 3: Use Scikit-Learn. We’ll use some of the available functions in the Scikit-learn library to process the randomly generated data.. Here is the code: from sklearn.cluster import KMeans Kmean = KMeans(n_clusters=2) Kmean.fit(X). In this case, we arbitrarily gave k (n_clusters) an arbitrary value of two.. Here is the output of the K …Clustering is the most popular unsupervised learning algorithm; it groups data points into clusters based on their similarity. Because most datasets in the world are unlabeled, unsupervised learning algorithms are very applicable. Possible applications of clustering include: Search engines: grouping news topics and search results. Market ...Second, global clustering criteria and unsupervised and supervised quality measures in cluster analysis possess biases and can impose cluster structures on data. Only if the data happen to meet ...PMC2099486 is a full-text article that describes a novel method for clustering data using support vector machines (SVMs). The article explains the theoretical background, the algorithm implementation, and the experimental results of the proposed method. The article is freely available from the NCBI website, which provides access to biomedical and …This study proposes an unsupervised dimensionality reduction method guided by fusing multiple clustering results. In the proposed method, multiple clustering results are first obtained by the k-means algorithm, and then a graph is constructed using a weighted co-association matrix of fusing the clustering results to capture data distribution ...Clustering: Clustering is the process of grouping similar data points, it is an unsupervised Machine Learning technique, and the main goal of an unsupervised ML technique is to find similarities ...Cluster headache pain can be triggered by alcohol. Learn more about cluster headaches and alcohol from Discovery Health. Advertisement Alcohol can trigger either a migraine or a cl...We also implement an SNN for unsupervised clustering and benchmark the network performance across analog CMOS and emerging technologies and observe (1) unification of excitatory and inhibitory neural connections, (2) STDP based learning, (3) lowest reported power (3.6nW) during classification, and (4) a classification accuracy of 93%. ...Clustering, the process of grouping together similar items into distinct partitions, is a common type of unsupervised machine learning that can be useful for summarizing and aggregating complex multi-dimensional data. However, data can be clustered in many ways, and there exist a large body of algorithms designed to reveal …May 30, 2017 · Clustering finds patterns in data—whether they are there or not. Many biological analyses involve partitioning samples or variables into clusters on the basis of similarity or its converse ... In microbiome data analysis, unsupervised clustering is often used to identify naturally occurring clusters, which can then be assessed for associations with characteristics of interest. In this work, we systematically compared beta diversity and clustering methods commonly used in microbiome analyses. We applied these to four …Learn about various unsupervised learning techniques, such as clustering, manifold learning, dimensionality reduction, and density estimation. See how to use scikit …Spectral clustering is a popular unsupervised machine learning algorithm which often outperforms other approaches. In addition, spectral clustering is very simple to implement and can be solved efficiently by standard linear algebra methods. In spectral clustering, the affinity, and not the absolute location (i.e. k-means), determines what .... In this paper, we advocate an unsupervised learFor visualization purposes we can reduce the data to 2-dimensions Clustering is the most popular unsupervised learning algorithm; it groups data points into clusters based on their similarity. Because most datasets in the world are unlabeled, unsupervised learning algorithms are very applicable. Possible applications of clustering include: Search engines: grouping news topics and search results. Market ... Introduction. K-means clustering is an unsupervised algorithm Unsupervised landmarks discovery (ULD) for an object category is a challenging computer vision problem. In pursuit of developing a robust ULD framework, … The commonly used unsupervised learning techniq...
Continue Reading